The water vapor distribution over the Arctic is poorly constrained in numerical models because in situ observations are scarce and current assimilation methods for satellite data render poor resolution in the initial condition. It is anticipated that improving the water vapor distribution both in the horizontal and in the vertical will have important consequences for improving weather forecasts over the Arctic in general and for improving forecasts of Arctic Cyclones in particular. The impact of water vapor gradients in the upper troposphere is anticipated to be an important driver for the formation of Tropospheric Polar Vortices (TPV’s), which in turn are known to trigger surface cyclogenesis. The presented work aims to repeat in the Arctic region the experiment conducted over the central Pacific, in which Transformed Retrieval’s (TR’s), derived from hyperspectral data (e.g., Migliorini 2012, Antonelli et al. 2017), and microwave data were both assimilated by WRFDA. The greatest impact of the data assimilation in the model initial conditions is found on the water vapor distribution (Antonelli et al. 2020). This study focuses on the work done to adapt the inversion system used, MIRTO, over the Arctic to generate data for a 3-day assimilation cycle during which an Arctic Storm formed at the end of July 2020 (Fig 1).
Fig. 1: Satellite mosaic of true color NPP/NOAA 20 images of the Arctic on 28 July 2020 (courtesy of NASA WorldView) Fig. 2: Extent of Ice Loss (27 July 2020) with respect to median ice edge between 1981 and 2010. Courtesy of National Snow and Ice Data Center – NASA Earth Observatory
The 2020 Arctic cyclone is an interesting case not only for the cyclogenesis and subsequent ice loss, but also for the challenges to the hyperspectral inversion algorithm caused by cloud cover and high latitude aerosols (Figs. 1-3). It requires new strategies for improving the cloud and aerosol masks used by the IR inversion system and to perform atmospheric profiling above clouds in overcast conditions. The goal is to better document the moisture and thermal structure of tropospheric polar vortices (TPVs) and Arctic cyclones, in the upper troposphere and at the surface, through assimilation of hyperspectral satellite data into regional models and through careful analysis of enhanced field experiment data sets (flight level, dropsondes, saildrones, etc).

Research has shown that the ice extent and thickness has a significant influence on the temperature and moisture fields near the surface in the Arctic. Therefore, in the continuation of the project, the effort will focus on improving the retrieval capabilities in presence of cloud contamination, which presents a special challenge to hyperspectral retrieval algorithms, with the overall goal of improving the lower boundary condition and thermodynamic structure in the troposphere in both WRF and COAMPS over the Arctic region.
CrIS and IASI over the Arctic
In the relatively data-sparse Arctic, the combined coverage of polar orbiting satellites is comparable to that of a geostationary satellite, and represents a rich source of information to be used by models and by scientists. In a 2 hoursperiod CrIS and IASI provide more than 120,000 observations with spatial and temporal coverage similar to what
geostationary spacecrafts provides at lower latitudes (Figs. 4-6). Timely ingestion of such a large volume of observationsin NWP models can be achieved if spectral inversion is performed off-line of NWP model runs.
Resulting Level 2 data(retrievals), which are highly compressed forms of the observed spectra, are then assimilated instead.
Fig. 4: CrIS (NPP-J01) Fig. 5: IASI (METOP-A,B,C) Fig. 6: CrIS (NPP-J01)
and IASI (METOP-A,B,C)
MIRTO – the hyperspectral data inversion system
The Space Science Engineering Center, in collaboration with the Mauna Kea Weather Center of the University of Hawaii has developed a regional retrieval processor for high spectral resolution infrared data (Fig. 7). The core of the processor makes use of a bayesian inversion system, hereafter referred to as MIRTO, which combines a-priori knowledge of the atmospheric state, based on available Numerical Weather Prediction forecasts, with physical information embedded in satellite observations.
Forecasted temperature and water vapor mixing ratio fields are adjusted to produce synthetic radiances closer and closer to the Soumi-NPP CrIS and METOP IASI observations.
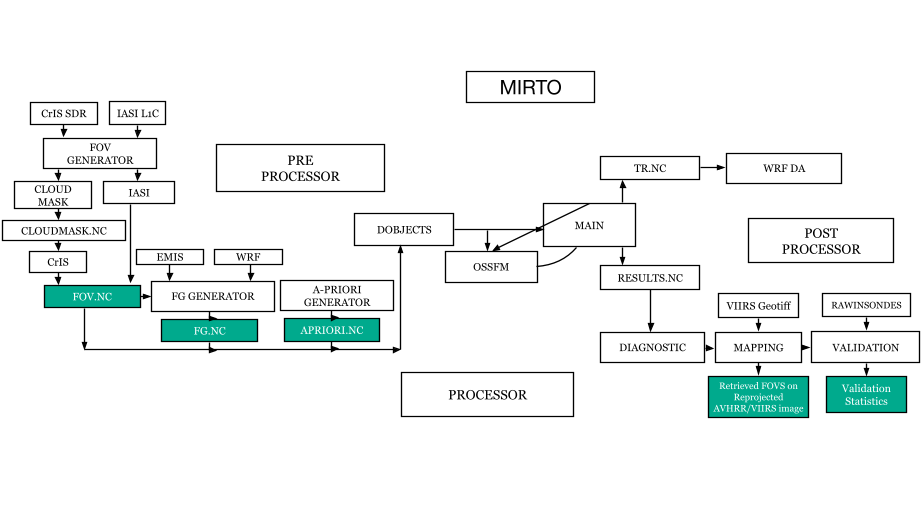
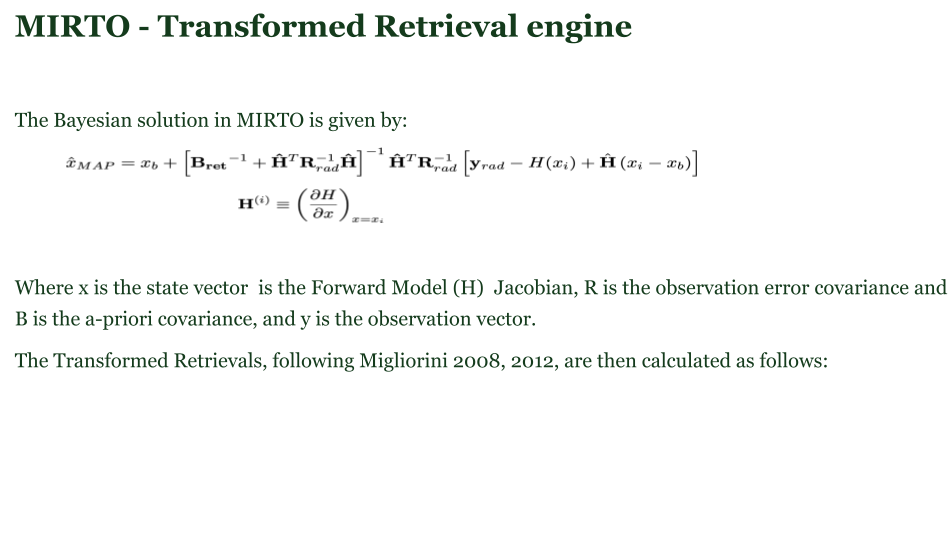


MIRTO – the assimilation scheme
The TR are fed to the NWP system in use at the MKWC of the University of Hawaii (Fig. 8). The system is based on a prognostic (WRF-DA) and a diagnostic (WRF) module and it is run in Rapid Update Cycle mode every 6 hours, with a full re-initialisation (cold start) done every 3 days. WRF/WRFDA are run in 6h-cycling mode using the previous cycle forecast as a background and assimilating: Surface, maritime, aircraft and radiosondes observations (MADIS database, NOAA); and Transformed Retrievals from hyper-spectral InfraRed satellite observations, produced locally from satellite data (Fig. 9).


Application over the Pacific
The paucity of in situ observations over the central North Pacific area and the highly homogeneous background presented by the ocean, provide a good opportunity to test the efficacy of this hyper-spectral data inversion system. Nearly real-time (less than 60 minutes from overpass time) web publication of retrieved atmospheric profiles is made possible by the availability of a Direct Broadcast system that provides data from the Suomi-NPP/NOAA20 platforms (CrIS and VIIRS) and from METOB A/B/C platforms (IASI and AVHRR) (Fig. 10). The main goal for the implemented MIRTO system is to feed the WRF forecasting system used at the MKWC of the University of Hawaii with assimilation-friendly data.

Satellite retrievals exploit the information content embedded in thousands of channels provided by hyperspectral sensors. Their assimilation into NWP models represents a gain in computational efficiency over current radiance assimilation methods. MIRTO implements the Migliorini theoretical approach (Migliorini et al., 2008) to feed a limited-area weather RUC modelling system (Fig. 11). The Migliorini approach provides a framework to effectively remove the a-priori information from the retrievals, and it is independent of the characteristics of the hyperspectral instruments. The results of the data assimilation experiments performed confirm the validity of Migliorini’s approach and show how the transformed retrievals (TR) derived from two different hyperspectral instruments are treated in exactly the same way by the assimilation system.

The results confirm that over the Central North Pacific region the temperature field is in general well predicted, thanks to the assimilation of conventional observations while, the water vapor field is less well constrained. Therefore, a larger impact of the assimilation of hyperspectral data is seen in the distribution of water vapor in the resulting analyses (Fig. 12). Similar results are expected over other regions of the planet such as the Arctic.

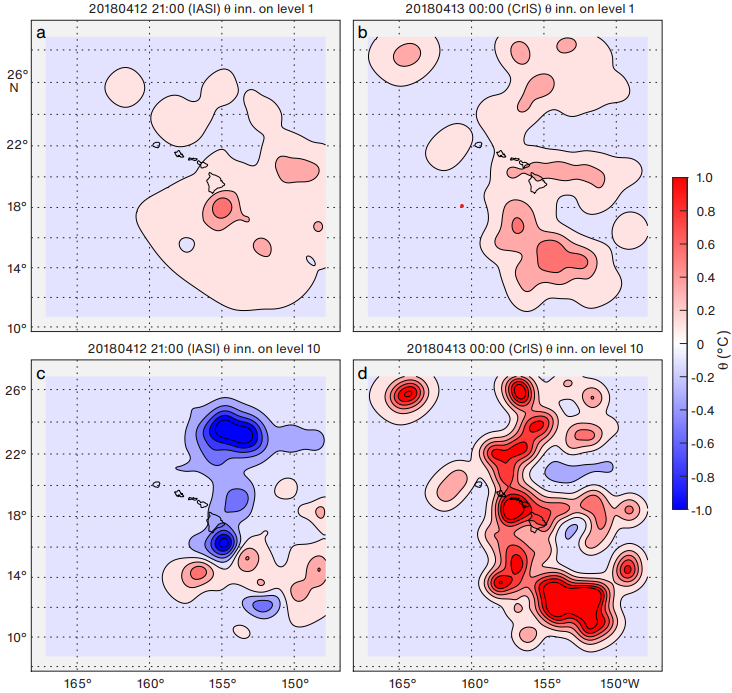
Application over the Arctic
MIRTO has been adapted to work in the Arctic Region primarily by modifying the surface emissivity database. Like for the Pacific setup, it uses the WRF forecast as first guess and it has been applied to CrIS and IASI data. The optimal time coverage of the available satellites allows MIRTO to produce about 1500 clear-sky convergent retrievals within a 2 hour period (statistics taken between 23:00 and 01:00 UTC over the region between 75 and 90 degrees North) (Fig 13 and 14).
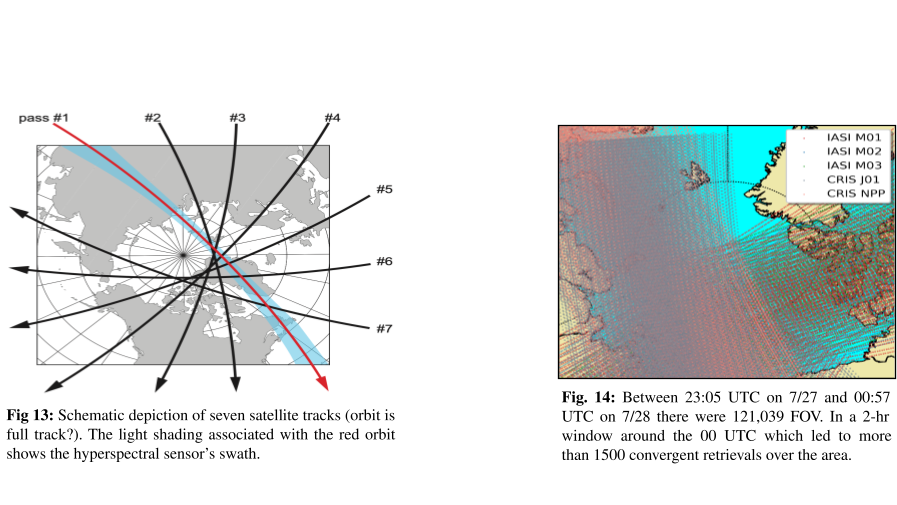
Figure 15 shows the consistency of CrIS retrievals obtained from two consecutive overpasses (at 11:21 and 12:09 UTC of the 27th July 2020). In both cases MIRTO captures the temperature inversion at 800 hPa and it diverges from the rawinsonde only near the surface as the selected FOVs (red ellipses) are over ice and water, while the rawinsonde station is over bare soil, which causes air temperature near the surface to reach 18° Celsius.
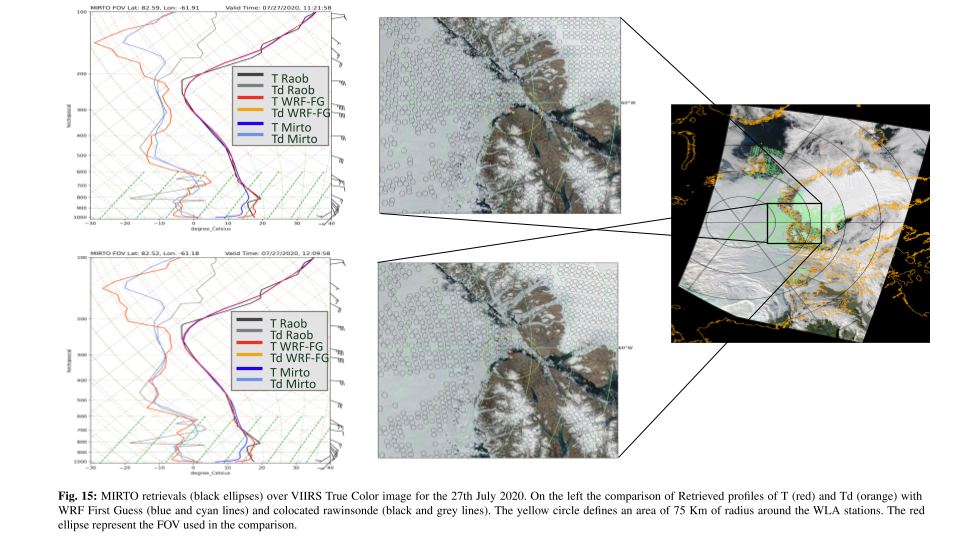
The TR are fed to the NWP system in a similar fashion to the one in use at the MKWC of the University of Hawaii. The systems is based on a prognostic (WRF-DA) and a diagnostic (WRF) modules and it is run in Rapid Update Cycle mode every 6 hours, with a full re-initialization (cold start) every 3 days. Setup of the model has been completed, and first tests have been performed. In the upcoming months, 3 days worth of CrIS and IASI TRs will be assimilated for the time period 27-29 July 2020, and the impact of the TR over the Arctic will be assessed by comparing the model output to a control run.

Status and Issues over the Arctic
Cloud cover over the Arctic presents a special challenge to hyperspectral retrieval algorithms, which work optimally in clear sky conditions. For the 3 day period under consideration the number of observations in clear sky conditions (less than 5% of cloud contamination in the FOV) are ten times smaller than the observations in overcast conditions (more than 95% cloud contamination) (Fig. 18). The ability to invert the hyperspectral data above overcast FOVs would therefore represent a significant increase in the atmospheric information fed to the NWP models.
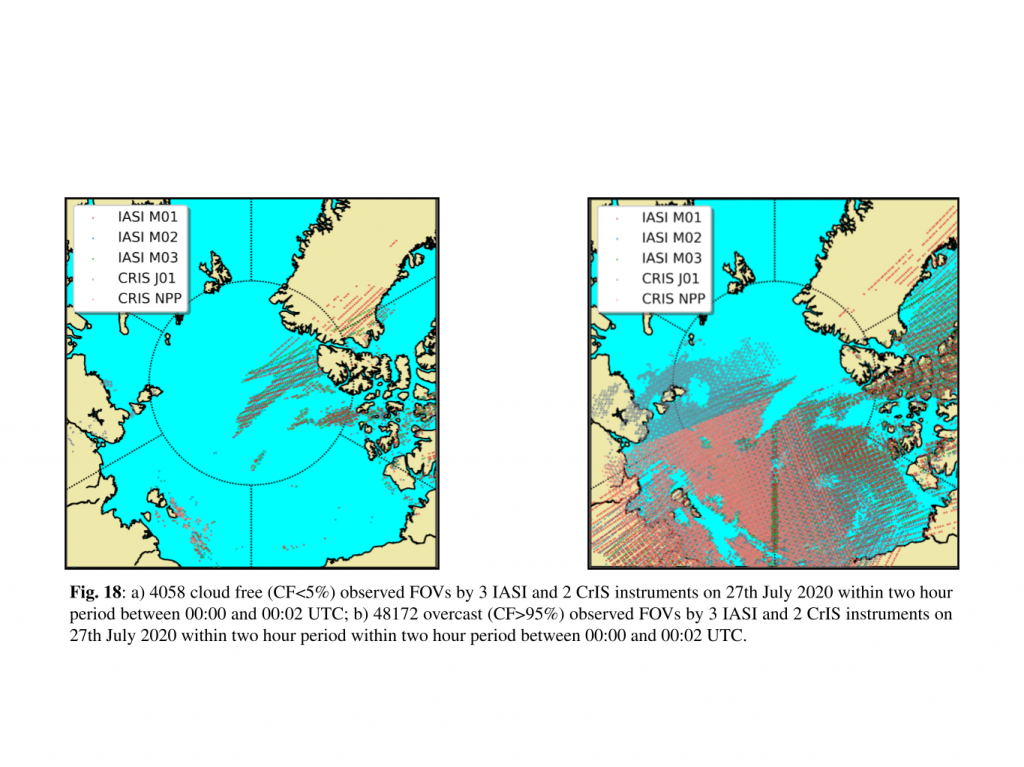
The proposed strategy is to develop and refine an approach for atmospheric profiling in cloudy conditions. The idea is to conduct hyperspectral retrievals above cloud top for overcast scenes, and to enable coupling of these retrievals with microwave observations in the assimilation system to gain the ability to penetrate clouds. The complementary nature of these satellite data sets, available from instruments flying together on polar orbiting satellites, has produced verifiable improvements in the water vapor distribution in data assimilation tests over the Pacific. Retrievals in overcast conditions are expected to be feasible by adding the surface pressure to the retrieved state vector and by including cloud emissivities in the surface emissivity database used by MIRTO. More complicated cases could arise in partially cloud contaminated FOVs which might not be properly classified by the VIIRS cloud mask. Figure 19shows one of these cases. The interesting and surprising aspects of partially contaminated FOVs now under investigation in the Arctic Region is that MIRTO picks up the condensation level where Td (in orange) crosses T (in red), and still provides an accurate retrieval above the cloud.

A great deal of work has to be done to properly characterize the atmosphere in presence of clouds, especially for partially contaminated FOVs. In this case, besides the addition of Microwave data, it would be interesting to explore the NOAA cloud cleared radiances products available for CrIS observations.
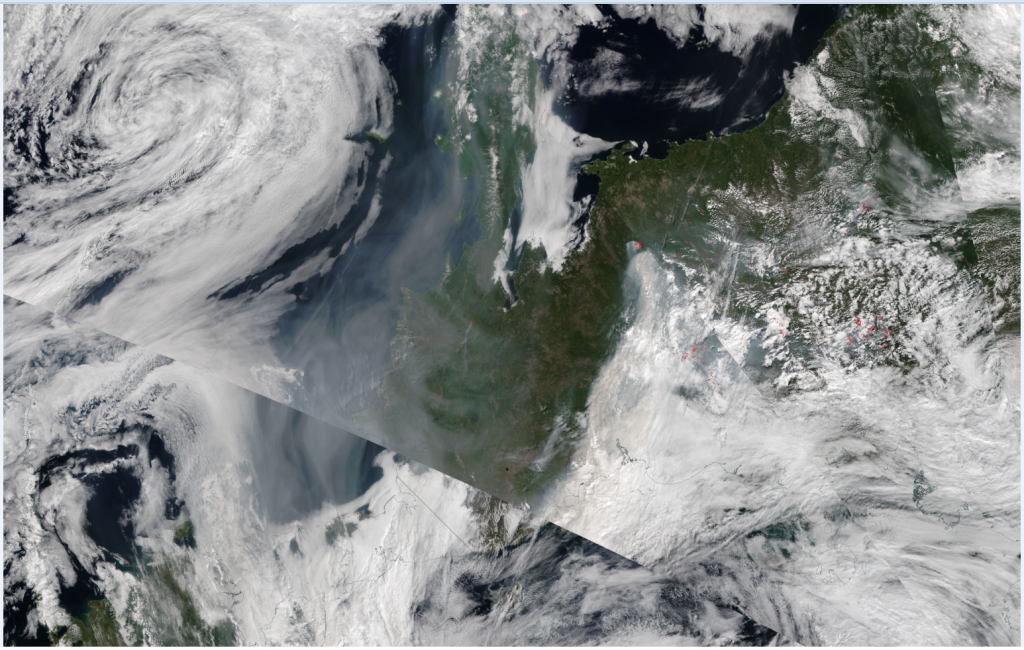
images of the Arctic on 28 July 2020 with smoke
contamination due to Siberian Fires. (courtesy of NASA
WorldView)
Finally, using the colocation tools developed for CrIS and VIIRS, it is also anticipated that a second improvement in the assimilation approach is possible based on the implementation of an aerosol mask, so that areas with significant smoke from wildfires can be flagged, and potential aerosol contamination of the TR’s can be monitored and investigated. Observational data collected by aircraft during the 2022 CNRS Field Experiment, will provide invaluable information with which to validate the accuracy of our retrievals and data assimilation approaches and to investigate our hypotheses regarding the energetics of Arctic Cyclones.
References:
Antonelli, P., Cherubini, T., Businger, S., de Haan, S., Scaccia, P., & Moncet, J. (2020). Regional Assimilation System
for Transformed Retrievals from Satellite High-Resolution Infrared Data, Journal of Applied Meteorology and
Climatology, 59(7), 1171-1193. Retrieved May 21, 2021, from https://journals.ametsoc.org/view/journals/apme/59/7/
jamcD190203.xm
Antonelli, P., and Coauthors, 2017: Regional retrieval processor for direct broadcast high- resolution infrared data.
Journal of Applied Meteorology and Climatology, 56 (6), 1681–1705, doi:10.1175/JAMC-D-16-0144.1, URL https://
doi.org/10.1175/JAMC-D-16-0144.1, https:// doi.org/10.1175/JAMC-D-16-0144.1.
Migliorini, S., 2012: On the equivalence between radiance and retrieval assimilation. Mon. Wea. Rev., 140 (1).
Migliorini, S., C. Piccolo, and C. Rodgers, 2008: Use of the information content in satellite mea- surements for an
efficient interface to data assimilation. Monthly Weather Review, 136, 2633– 2650.
Cherubini, T., S. Businger, and R. Lyman, 2011: An operational perspective for modeling optical turbulence. Seeing
Clearly: The Impact of Atmospheric Turbulence on the Propagation of Extraterrestrial Radiation, S. Businger and T.
Cherubini, Eds., VBW Publishing, 165–182.
Contacts:
paolo.antonelli@adaptivemeteo.com, paolo.scaccia@adaptivemeteo.com,
tiziana@hawaii.edu, businger@hawaii.edu
Acknowledgments:
We thank the SSEC and the MKWC for supporting the implementation of the regional retrieval system based on Mirto.
This research was supported by the Office of Naval Research under ONR Award N00014-18-1-2166.